How much time could your small business save with smarter tech?
For many small businesses, keeping up with day-to-day operations is overwhelming enough—leaving little time for innovative solutions. Yet, new technologies like Retrieval Augmentation Generation (RAG) models offer ways to work smarter, not harder, by helping teams tap into the power of large language models (LLMs). But what exactly are RAG models, and how can they help your business?
RAG combines the efficiency of real-time information retrieval with the intelligence of advanced language generation. Imagine giving your team the ability to make faster decisions, provide instant customer responses, or streamline complex internal processes—all backed by the latest data. Originally designed for large enterprises, these cutting-edge tools are now accessible and scalable for small businesses too.
In this article, we’ll take a closer look at how RAG models enhance decision-making, improve operations, and offer a competitive edge for small teams. Ready to explore how these innovations can propel your business forward? Get in touch and let’s talk about solutions designed for your unique needs.
By the end of this guide, you’ll know how to implement RAG models in your operations and optimize your efficiency.
Unlocking the Power of Retrieval-Augmented Generation (RAG) for Small Businesses
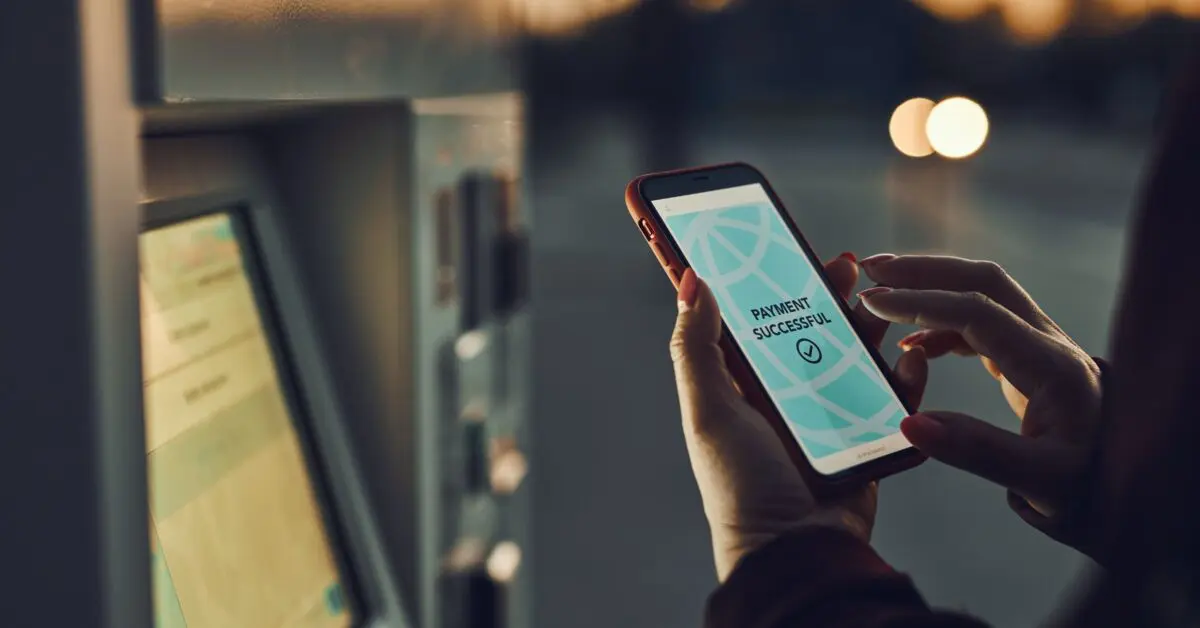
Imagine having an AI assistant that not only finds relevant information within seconds but also uses that information to create valuable insights for your business. That’s the potential of Retrieval-Augmented Generation (RAG). By combining retrieval-based methods with the latest generative AI models, small businesses can tap into powerful data-driven solutions to solve real-world challenges swiftly and efficiently.
What is RAG, and Why Does It Matter for Small Businesses?
RAG models are AI-driven systems that retrieve pertinent data from diverse sources and generate human-like outputs. This process enhances decision-making by providing contextual, relevant data during conversations or tasks. For small businesses, this could mean faster decision-making, personalized customer service interactions, or better-targeted marketing.
Where typical large language models (LLMs) stumble by producing inconsistent or broad results from vast datasets, RAG models shine. They provide focused, relevant information. RAG’s dual capability bridges the gap between data retrieval and actionable AI insights, producing outputs that closely align with your specific business needs.
How RAG Enhances Your Business Decision-Making
A key advantage of implementing RAG models lies in improving business decision-making. When closely integrated into operations, RAG-driven tools can:
Streamline data analysis: Imagine condensing hours of market research into minutes. RAG systems scan extensive databases to offer insights that can help in assessing industry trends, competition, and customer sentiment. Companies using similar strategies saw a 25% sales increase when applying AI-powered product recommendations.
Optimize time-sensitive processes: Small enterprises often face data-overload problems. RAG helps automate data extraction from internal systems like sales reports or customer emails. The improved response time fosters quicker decision cycles, enabling companies to react faster to market shifts.
Boost Business Intelligence: Being able to identify actionable insights from within vast amounts of raw data is a serious challenge. But with RAG-based systems, companies can identify trends, manage risk, or even engage in predictive analytics to stay ahead of competitors.
RAG in Marketing & Customer Service for Small Teams
By embedding RAG models into customer-facing systems, small businesses are able to elevate marketing strategies and customer service levels. Here’s how:
- Personalized automation: RAG allows small businesses to create personalized marketing campaigns similar to large corporations. For instance, by automatically analyzing customer preferences, RAG can generate tailored product recommendations and enhance customer experiences—as demonstrated in the retail sector’s successes with predictive algorithms.
24/7 Customer Support: Small businesses often lack the workforce to run 24/7 customer service. With RAG-driven bots, this becomes feasible, ensuring that customers receive “smart” help even outside business hours. Whether it’s FAQs, product support, or personalized recommendations, RAG provides precise and non-generic responses.
Campaign Efficiency: Small businesses have maximized ROI by adopting AI-enhanced campaigns. Additionally, SMEs are able to improve marketing effectiveness significantly by tying channels like SMS and social media together through RAG-supported omnichannel campaigns.
Implementing RAG in Business: A Practical Guide
To make RAG work for your small business, you’ll need a clear plan to get started. Here’s a step-by-step process on how you can embed Retrieval-Augmented Generation within your systems:
Identify Key Use Cases: Before leveraging any AI model, it’s essential to pinpoint specific areas where RAG will add value. For example, small retail businesses might use RAG for real-time customer support, while a consultancy might deploy it for quick contract generation.
Choose the Right Tools: Skipping proprietary solutions for open-source tools like Hugging Face Transformers or cloud-based APIs such as OpenAI, Gemini or Antropic can ease scaling without hefty costs or complex onboarding processes.
Run Pilot Projects: Start small. Implement RAG models in one part of your business, test them, and measure their impact by analyzing how much time, resources, or costs were saved. As noted in various success stories, businesses observed major improvements when tackling one pain point at a time.
Integrate with Existing Workflows: Ensure smooth integration of RAG with current tools such as marketing automation and CMS platforms. Many RAG models come as plug-and-play solutions, reducing technical complexity involved in integrating them into your current systems.
Monitor & Adjust: With AI tools, continuous learning is key. Incorporate regular feedback to fine-tune the models and make sure they are producing accurate results.
Top Benefits of RAG for Small Businesses
Retrieval-Augmented Generation offers tangible benefits that go far beyond the theoretical. Here are a few ways small businesses can directly benefit from adopting this technology:
Reduced Operational Costs: By employing RAG-backed automations, businesses can drastically cut down on personnel required for data-heavy tasks. As companies face rising wage expectations, RAG can support their ability to stay lean while remaining operationally competitive. For example, SMEs engage capable AI tools for personalized marketing without having to invest in additional technology talent.
Improved Customer Retention: When customers receive quick, relevant responses, it improves their overall experience, leading to greater customer loyalty. Research highlights that personalized engagements drive both satisfaction and repeat purchases.
Faster Time-to-Market: Small businesses with RAG technology can act quicker by efficiently transforming data points into actionable strategies. These advantages are especially crucial when pursuing fast-moving opportunities in competitive landscapes.
Leveraging RAG for small businesses opens doors to unprecedented efficiency and growth. By tapping into rich, relevant data and using AI to personalize operations, small teams can now punch well above their weight. Stay tuned for the next stage, where we address the tangible challenges faced by SMEs in adopting RAG, and offer solutions that ensure a smoother implementation.
Talk to our team on how RAG and LLMs can help your business explore new capabilites that make a dramatic difference. Start by talking about what you want, you might be surprised.
How RAG Models Enhance Decision-Making in Small Enterprises

In an era where every decision can impact revenue, speed and accuracy have become essential weapons for small businesses. Retrieval-Augmentation Generation (RAG) models, powered by Large Language Models (LLMs), offer an exciting opportunity for small enterprises to make data-driven decisions. By leveraging vast amounts of unstructured data, RAG models digest and present insights that can lead to smarter, faster decisions, particularly in business-critical areas like customer relationships and market adaptability.
Extracting Actionable Insights from Unstructured Data
RAG models can process vast quantities of unstructured data—such as customer feedback, emails, and social media posts—and convert it into actionable insights. This is crucial because 90% of business data is unstructured, yet it holds valuable information that can enhance decision-making.
By applying RAG to customer feedback, a small business can quickly analyze recurring customer pain points and shift their product strategy accordingly. For example, a small coffee shop could analyze online reviews to identify the most requested menu additions, making data-backed decisions in real-time.
“RAG models can transform previously unusable blocks of text into well-organized insights, significantly boosting business intelligence,” highlights Yasmin Wang.
Enhancing Market Adaptability through Faster Decision Loops
Time is often a scarce resource for small businesses, and staying flexible in a fast-changing market is essential. RAG models help to close decision-making loops quickly, allowing businesses to swiftly adapt to new market conditions based on immediate data assessment.
A small business that sells retail products, for instance, can benefit from implementing a RAG model to analyze purchasing trends. By quickly identifying which items are gaining popularity, they can redirect marketing resources toward those high-demand products, ensuring they stay competitive without incurring large investment costs.
Companies using AI-enhanced models like RAG saw reduced lag times between data collection and execution, improving their market adaptability by up to 40%. – Sofia Fernandez
Supporting Strategic Decisions with Data Transparency
Decisions backed by data and transparent reasoning lead to more confidence in execution. One challenge small businesses often face is decision fatigue—feeling overwhelmed by choices without clear guidance on which move will yield the best outcome. RAG models solve this by not just providing data, but giving rationale behind each recommendation, leading to clearer strategic decisions.
A practical example is how small logistics companies are using RAG models to explore multiple shipping routes, where the AI-driven system clearly explains why one route may be faster or more cost-effective, giving the manager both options and the rationale.
“By integrating RAG models with business intelligence tools, small enterprises are able to make pivotal choices that are transparent and backed by hard data.” – Yasmin
Reducing Cognitive Load and Avoiding Burnout
Decision-making is mentally taxing, especially for small business owners juggling multiple roles. With RAG models, that cognitive load is distributed by offering pre-digested insights, allowing business owners and decision-makers to focus on creative and strategic aspects of their business.
For example, instead of manually sorting through hundreds of customer inquiries, a small business could implement a RAG model to filter priority issues, offering suggested responses or flagging the most immediate issues to deal with.
Outsourcing this mental load helps avoid burnout, a common challenge in the small-business world where 78% of SMEs report facing productivity drops due to workforce fatigue.
RAG models are revolutionizing decision-making for small enterprises by providing clarity, reducing decision-time, and aligning strategies with real-world data. As these models become increasingly affordable and accessible, businesses of all sizes can leverage this technology.
Ready to take concrete steps toward transforming your decision-making process with RAG? Discover the actionable power of LLMs today!
Step-by-Step Guide: Implementing RAG LLMs in Your Business Operations
Retrieval-Augmented Generation (RAG) models, powered by large language models (LLMs), offer small businesses a unique toolset to make data-driven decisions. But, unlocking their full potential requires a structured approach that aligns with your existing business processes. Let’s walk through a pragmatic step-by-step guide on how to implement RAG LLMs in your daily operations.
Identify the Key Areas Where RAG Will Have the Most Impact
The first step in leveraging RAG LLMs is to assess which areas of your business could benefit the most from AI-driven insights. Determine if you aim to boost customer service, automate workflows, or improve data analysis.
- Customer Service: RAG models can power chatbots, allowing small businesses to deliver 24/7 customer support, at a fraction of the cost of hiring additional staff. A practical example is a small e-commerce store that increased customer retention and satisfaction by 30% after implementing RAG-driven chatbots.
- Content Creation and Marketing: Many small teams find it challenging to keep up with content creation. RAG models can simplify this by generating high-quality blog posts, social media content, or email newsletters, thus saving time.
- Data Insights and Business Intelligence: Collect feedback, extract sentiments, and analyze reviews using LLMs. In one case study, a retailer applied LLMs to understand customer pain points, resulting in a 25% increase in sales after optimizing product recommendations.
Select the Right RAG Tools and LLM Providers
Choosing the right tool for your specific business needs is critical. Not all LLMs and RAG models are created equal—and they shouldn’t be viewed as plug-and-play.
- Budget and Scale: Small businesses should consider both the accessibility and affordability of their options. Popular open-source models, like Hugging Face Transformers, offer flexibility at a lower cost, while commercial platforms like Google Cloud AI provide extensive support but may incur higher fees.
- Feature Comparison: Each platform provides unique features. For example, platforms like OpenAI API offer a range of pre-trained models and provide robust integration options. By carefully evaluating the match between your existing tools and the model’s integration capabilities, you can make a seamless transition.
Prepare, Preprocess, and Fine-Tune Your Data
Effective implementation of RAG LLMs comes from training your models on relevant, high-quality data. Small businesses may find themselves lacking vast datasets initially, but this doesn’t mean optimal results aren’t possible.
- Data Collection and Cleaning: Start with gathering relevant information—whether it’s customer data or research archives. However, ensure it’s clean and ready to use. Models trained on inaccurately processed data can skew outputs.
- Leverage Fine-Tuning Capabilities: Even pre-trained models benefit from fine-tuning. This step aligns the LLM with your needs, improving accuracy when processing unique terminology relating to your industry.
A popular technique employed by smaller firms is transfer learning, which saves time by using pre-trained models that can be customized with minimal specific data. Leveraging Large Language Models for Small Business Success emphasizes how this approach cuts costs on full retraining, aligning output to localized business needs.
Seamlessly Integrate the Model Into Existing Workflows
Once you’ve fine-tuned your RAG models, the next step is integrating them with your daily workflows. Look beyond just “fitting” the model into your system; focus on minimizing friction for your team.
- User-Friendly Dashboards: Ensure that the interface you integrate is easy to navigate. For small businesses with limited technical know-how, plug-ins that work with existing CRMs, like Salesforce or HubSpot, can ease the complications.
- Automating Repeated Tasks: Set up automated responses for low-complexity customer queries or allow the system to triage support tickets. This will expedite responses and give your team more bandwidth for higher-priority work.
Ensure Ongoing Performance Monitoring & Model Maintenance
Deploying RAG models isn’t a “set it and forget it” process. You need to establish performance checkpoints to make adjustments as necessary.
- Regular Audits for Accuracy: Monitor how well your model meets expected performance benchmarks. For instance, sales teams should conduct biweekly reviews to evaluate RAG-generated product recommendations’ effectiveness.
- Ethical Oversight: LLMs can introduce bias into recommendations or outputs if they’re unchecked. Make it a point to regularly review outputs and intervene as necessary, ensuring a human officer is always on board for major decisions.
Optimize and Tweak
Always loop back and adjust your approach. As your business grows, so will the way your RAG models should operate. Tailored fine-tuning over time keeps the models aligned with evolving business goals.
By understanding the full process, you ensure that implementing RAG technology is not an overwhelming undertaking but rather a strategic advantage perfectly suited for small businesses. Now that you’ve explored the implementation process, you may be ready to learn more about the broader conversation around overcoming common challenges that arise with adopting RAG LLMs.
Top Benefits of Using Retrieval Augmentation Generation for Small Teams
Small teams in businesses juggle multiple tasks daily, often with constrained resources. Leveraging innovations like Retrieval Augmentation Generation (RAG) tied with Large Language Models (LLMs) can bridge gaps and make these challenges manageable. By infusing AI-powered solutions, small business teams can streamline operations, boost problem-solving capacities, and gain a competitive edge without breaking the bank. So, how exactly does RAG give these teams an advantage? Let’s dive into the details.
1. Boosting Productivity and Saving Time
RAG models significantly reduce the amount of manual data searching and decision-making. Instead of sifting through vast amounts of data, RAG sifts through relevant, pre-processed databases, delivering key pieces of information to the user’s fingertips in seconds. This allows small teams to focus on core operations.
A stunning example is LLMs facilitating content creation: RAG can generate SEO-ready blogs, product descriptions, and marketing materials within a fraction of the time it typically takes humans, saving both manpower and operational latency.
“Small businesses can cut up to 50% of their content creation time by automating processes with LLM-integrated tools,” notes a recent Gartner report on SME automation.
2. Enhanced Decision-Making with Real-Time Data
No more relying on outdated sheets and gut feelings. Small teams can make well-informed decisions powered by instantaneous, AI-enhanced insights. RAG models are capable of pulling live information from multiple sources, analyze trends, and generate summaries or next-best-action recommendations relevant to the team.
Whether it’s analyzing customer behavior or formulating pricing strategies, these AI solutions ensure decisions are backed by data. A small e-commerce site, for instance, boosted their sales by 25% using RAG-powered recommendation systems, while also reducing the number of returns with more accurate product matching.
For small teams, the power of real-time business intelligence tools ensures faster decision-making and pivots to new opportunities.
3. Personalizing Customer Interactions
Ever wonder how small businesses compete with bigger brands when it comes to customer service? RAG empowers small teams to deliver personalized experiences by analyzing customer input and interactions. AI-driven chatbots leveraging RAG technology can answer customer queries 24/7, recommend products, and even pull information from user reviews to correctly assess customer sentiment.
This kind of customer care leads to higher satisfaction and engagement levels. LLMs in small businesses successfully automate routine service tasks and transform customer queries into actionable insights without additional staffing, offering low-cost but high-impact solutions.
“With AI automation, small businesses see a whopping 70% increase in the efficiency of customer service responses,” a post from ITPro.com suggests.
4. Cost-Effective Workflow Automation
Small organizations face budget constraints typical of their size, which means they need tools that deliver large-scale impact at a low cost. RAG-driven solutions provide significant workflow automation without straining resources. Tasks that typically require human input—such as sorting customer feedback, classifying documents, and automating follow-ups—are now executed nearly seamlessly through an AI-powered backend.
Additionally, No-code AI solutions offer a cost-effective method for scaling systems, enabling small teams to manage critical operations without the need for extensive programming skills.
For example, tools like OpenAI API or Google Cloud’s AI tools reduce the need for expertise in complex data tasks while cutting operational costs by almost 40 percent through efficient decision-making tools. Teams can deploy systems faster, reduce redundant labor costs, and foster constant improvements across operational workflows.
5. Reducing Human Error Through Automation
Small layoffs or manual operational loads often introduce additional risk and human error. Miscommunication, calculation errors, or missing documentation are more likely when workload outweighs the resources available. By integrating RAG with LLM-powered technologies, procedures like data entry, market trend analysis, and order follow-ups are automated.
This level of automation drastically minimizes mistakes, allowing small teams to focus on high-brow critical tasks that require human intervention. Moreover, with frequent updates in AI tools and natural language models improving periodically, the team wouldn’t exceed capacity—you’ll always have enough resources to handle any scenario with punctual reliability.
A report from McKinsey highlights that businesses incorporating AI-driven automation see a reduction of errors by 20% across repetitive task areas, meaning teams become more reliable and efficient with less oversight.
RAG models empower small businesses to accomplish tasks more quickly, operate effectively, and scale, allowing them to compete with larger enterprises. But this innovation also comes with specific considerations for implementation. Up next, we’ll outline several common obstacles small businesses face when adopting RAG technology and actionable steps to overcome these challenges. No need to stress—all of this is manageable on even the smallest budget!
Overcoming Common Challenges: Tips for Successfully Adopting RAG LLMs
The power of Retrieval-Augmented Generation (RAG) Large Language Models (LLMs) is becoming evident across various industries, including small businesses. But despite their potential, many companies encounter hurdles when implementing this technology into their workflow. By addressing these challenges head-on, small teams can turn obstacles into stepping stones for growth. Below, we explore the most common challenges and how your small business can successfully adopt RAG-powered LLMs.
Challenge 1: Data Quality and Preparation
High-quality data is the backbone of any AI model. Poor data can lead to inaccurate results, frustrating users and wasting resources. Reports show that data challenges account for a major portion of AI project failures. In a survey from Infosys and LTIMindtree, nearly 50% of respondents cited data quality issues, including security concerns.
To avoid these pitfalls:- Clean and pre-process your data regularly to remove any inaccuracies and missing values.- Segment your data carefully to ensure you’re feeding the most relevant information into your RAG LLM system.- Ensure compliance with regulations like GDPR to protect your data and avoid legal complications.
Prioritizing these steps for data preparation can drastically improve model accuracy and empower your team with high-value insights.
Challenge 2: Integration with Existing Business Tools
Integrating a complex language model like RAG into your business’s tech stack can sound intimidating. Seamless integration with existing workflows is crucial for reaping the full benefits. Poor integration can cause functional disruptions that slow down operations.
An effective method is to start with small and defined tasks before scaling up. According to a report by groSamriddhi, small businesses have successfully enhanced workflow automation by focusing on specific use cases such as content creation and customer service.
Additionally:- Leverage API-based integrations for easier connections to existing platforms like CRM or ERP systems.- Use open-source tools like Hugging Face Transformers or cloud-based solutions from Google Cloud to manage integration seamlessly.
A hybrid approach that synchronizes AI efforts with your current platforms lowers the barrier and reduces disruptions.
Challenge 3: Training and Human Oversight
While LLMs can automate many tasks, human oversight remains crucial to avoid biased outputs or errors that can damage your reputation. Without vigilance, models may produce undesirable content or exaggerate facts, a phenomenon known as “AI hallucinations.”
To mitigate these risks:- Maintain human review loops to verify generated outputs before final use.- Conduct bias audits regularly on your dataset and model output, since biases can seep in through unbalanced data.- Provide adequate training so that your employees understand how to work in tandem with the LLM, including knowing when human judgment should override the machine.
As highlighted by this article, combining these practices helps harness AI’s potential while ensuring responsible usage.
Challenge 4: Addressing Cost and Accessibility Concerns
Budget constraints are often a limiting factor for small businesses, especially when experimenting with new technologies like RAG. Concerns about prohibitive costs prevent many enterprises from adopting advanced AI systems, but there are strategies to get around this.
Here are some budget-friendly approaches:- Use pre-trained models: By utilizing pre-trained models (available via platforms like OpenAI or AWS), you can reduce the need for extensive computing resources.- Collaborate with other small businesses to share resources and investment in AI tech. This means splitting subscription costs or pooling research data to improve model training.- Explore grant options or accelerator programs that specifically cater to small businesses integrating advanced AI technologies.
By tapping into affordable RAG solutions available today, small businesses can outweigh the initial costs and see a positive return on investment in performance automation, lead generation, and operational efficiency.
“LLM-powered applications proved to increase productivity by up to 15% for a small e-commerce firm, enhancing recommendation systems and workflow automation.” — Case study on implementing LLM
As you adopt RAG, these strategies should help offset many of the perceived financial barriers.
Challenge 5: Ethical and Privacy Concerns
It’s critical to consider the ethics and privacy implications of using RAG systems. AI is notorious for producing biased or incorrect data when improperly managed, which can lead to legal and reputational risks.
To ensure your RAG adoption is ethical and compliant:- Use a diverse dataset to train models and minimize algorithmic bias.- Implement strict privacy guidelines to secure sensitive business and customer data. Technologies such as encryption and secure API channels are must-haves.- Establish transparency in how AI systems are used within your business. Customers should know if an AI system is generating their product recommendations or customer service responses.
For compliance, ensure adherence to regulations like GDPR or CCPA, which govern data privacy and security. Following best practices in transparency also increases customer trust, a non-negotiable factor for small businesses.
Successfully integrating RAG LLMs may seem daunting, but through the strategic resolution of these challenges, small businesses can unlock AI’s transformative potential.
To move forward, take the next step by reviewing how large language models can streamline your business operations seamlessly. Read on!
Embracing RAG LLMs for Small Business Growth
Retrieval Augmentation Generation (RAG) models offer a tailored solution for small businesses to make smarter, more informed decisions. By integrating real-time data retrieval with generative AI capabilities, RAG models boost efficiency, optimize workflow, and create more personalized customer interactions. From decision-making enhancements to seamless implementation, this article has outlined the core advantages of adopting RAG technology.
The ability to harness large-scale data without needing a large team makes RAG especially powerful for small enterprises. As this technology continues to evolve, it will open new doors for automation and innovation, potentially changing how small businesses operate in the digital landscape. Now is the time to consider the benefits, overcome adoption challenges, and stay competitive in today’s business world.
Ready to explore how RAG can transform your business? Contact us today and discover the potential of this game-changing technology for your success.